TL;DR
Note: All use cases referenced in this blog are representative examples inspired by real-world patterns observed across industries. They are anonymized and generalized to protect confidentiality.
Legacy data services are buckling under the weight of modern business and technological demands. As enterprises lean into AI, real-time intelligence, and cross-functional collaboration, they must rethink their data strategies and infrastructure from the ground up. This blog unpacks the strategic shift from brittle pipelines to product-centric data foundations—emphasizing transformation in governance, organizational structure, and platform strategy.
Executive Summary
According to McKinsey, only 30% of digital transformations succeed—largely due to unclear data ownership, fragmented systems, and the absence of a strategic foundation. Companies that treat data as a strategic asset can outperform competitors by as much as 85% in sales growth.
Despite heavy investments in data technology, many enterprises remain hampered by fragmented systems, inconsistent metrics, and weak governance. The problem? A strategic flaw—treating data as a technical asset instead of a core business driver. Drawing inspiration from modern frameworks, this blog calls for a reimagination of data as a governed, productized capability aligned with enterprise objectives. With strategic insights and real-world case studies, we explore how visionary companies are rebuilding data foundations for scalability, trust, and intelligence.
"You don’t need more dashboards. You need a direction."
What Modern Data Strategy Is—and What It’s Not
Too many organizations treat data strategy as a box-ticking exercise—something to publish, not something to practice. In reality, a data strategy is a living framework that governs how data is captured, governed, activated, and measured for business impact. It’s not documentation. It’s operational DNA.
"A strategy is not what you write down. It's how you behave when systems break."
Let’s clarify what it’s not:
- Not a one-time document: Strategy must evolve alongside business and regulatory changes.
- Not a tech stack shopping list: Tools don’t make a strategy—alignment and accountability do.
- Not solely IT's responsibility: Data is a cross-functional concern, spanning business, product, legal, and engineering.
- Not just about compliance or reporting: Reporting is table stakes. Strategy unlocks competitive advantage.
A modern data strategy sets a vision for measurable business outcomes, accountability structures, and a roadmap for evolving capabilities.
Key Questions Before You Build a Data Strategy
Building a modern data strategy isn’t just about defining tools or target architectures. It starts by asking the right questions—the ones that cut across teams, clarify ownership, and anchor your work in real business value. These questions are foundational because they determine how your strategy will operate under pressure, scale with complexity, and stay relevant as your organization evolves.
Before diving into architectures or tools, organizations need alignment on foundational questions that shape direction, ownership, and value delivery.
- What business outcomes will this support? Strategy should be anchored in real initiatives—improving churn prediction, powering AI copilots, driving operational efficiency—not vague ambitions.
- Who owns stewardship, implementation, and measurement? Clear ownership avoids the chaos of shadow IT and misaligned expectations. This includes business data owners, technical leads, and governance.
- Where will governance live—and how will it be enforced? Governance can’t be an afterthought. It should be embedded in workflows—automated, enforced, and monitored.
- How do we ensure scalability across AI, real-time ops, and compliance? Your initial blueprint should anticipate future complexity, not collapse under it. This includes modular architecture and flexible contracts.
Answering these questions gives your strategy a spine.
Why Traditional Data Systems Fail
Understanding where traditional systems break down is crucial to designing something better. Many of today’s architectures are the result of decades-old assumptions: centralized control, waterfall planning, and infrequent change. But today’s enterprise needs real-time collaboration, regulatory readiness, and data agility baked into the system—not layered on top.
Legacy systems weren't built for collaboration, agility, or scale. They were designed for linear workflows and slow-moving requirements. In today’s world, they break down in familiar ways:
- Centralized governance that can’t scale: Approval queues turn into blockers, stalling delivery and encouraging teams to bypass the process.
- Ad hoc pipelines with no lineage: Spaghetti workflows result in unclear data flows, duplication, and no clear source of truth.
- Shadow IT and blurred accountability: Business teams build their own analytics stacks in silos, leading to governance risks and conflicting metrics.
"You can't solve strategic problems with patchwork pipelines."
This leads to the classic enterprise paradox: data-rich, insight-poor.
The Cost of No Strategy
Even with best intentions, many organizations operate without a coherent data strategy—often because they underestimate the consequences. But treating data as an afterthought rather than a foundational asset leads to systemic breakdowns. These aren’t just operational nuisances—they’re enterprise-wide risks.
When data is treated as a technical problem instead of a strategic asset, the entire enterprise suffers.
- Siloed systems: Each function builds its own pipeline, warehouse, and definitions, making alignment impossible.
- Conflicting KPIs: Different teams have different truths. Dashboards don’t match. Trust erodes.
- Regulatory exposure: Without visibility and control, compliance becomes a gamble.
- Infrastructure sprawl: Tools proliferate. Costs rise. Value lags.
It’s not just inefficiency—it’s decision paralysis at scale.
What an Effective Data Strategy Delivers
Creating a strong data strategy isn’t just about tools or architecture—it’s about unlocking tangible business outcomes. When done well, a strategic foundation transforms data from a liability to a competitive advantage. This section outlines the capabilities that mature data strategies consistently deliver—and why they matter to business leaders.
Use case: A global insurance provider implemented a productized data strategy, reducing the time to deliver risk dashboards from 3 weeks to 3 days, enabling real-time underwriting decisions.
A strategic data foundation doesn’t just support the business—it amplifies it.
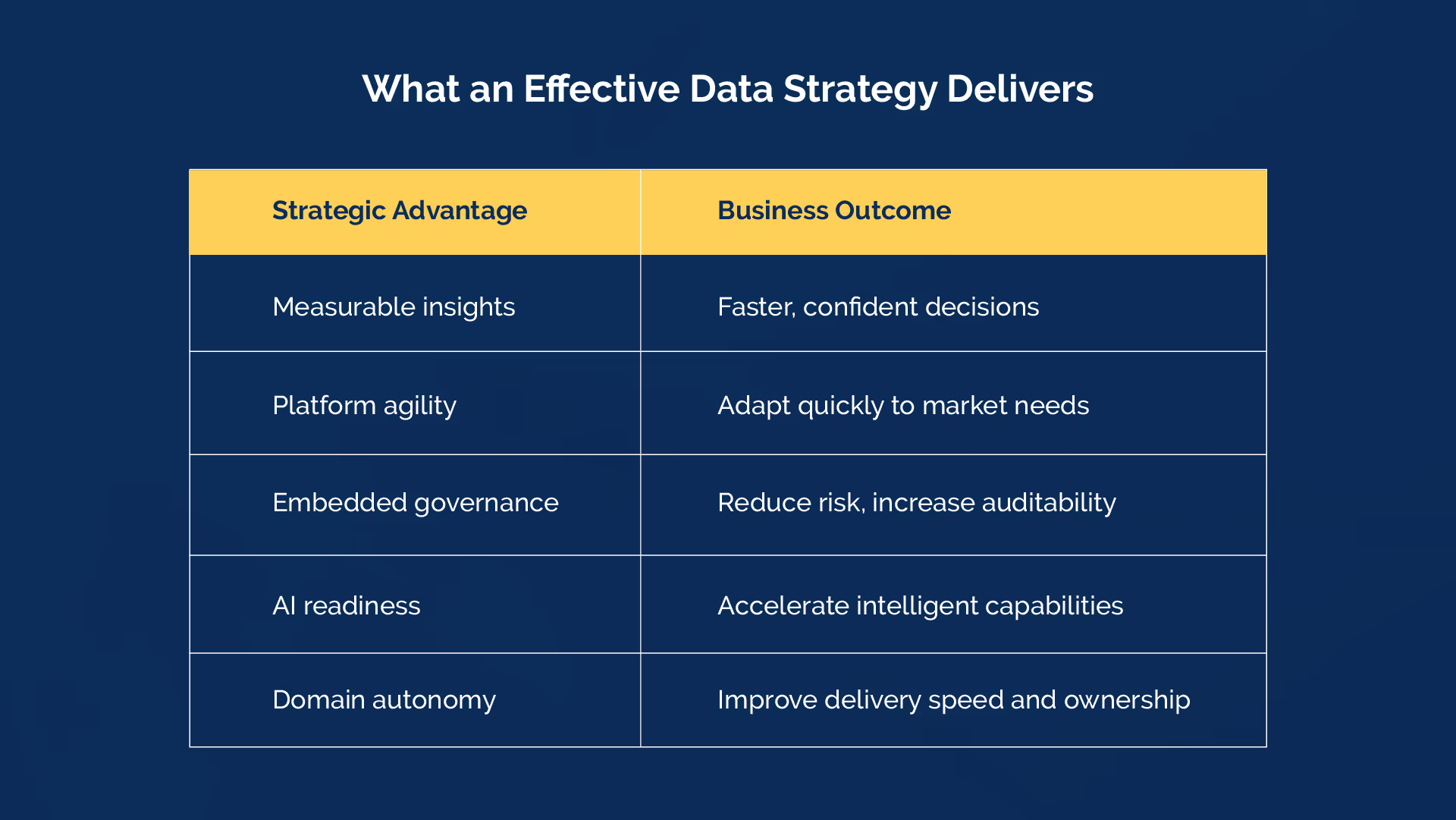
Aligning Data to Business Value
For data to deliver value, it must live closer to the decisions that matter. That means aligning data work directly with the priorities, rhythms, and accountability structures of the business. When done right, this alignment turns data from an operational utility into a strategic asset.
Use case: A fintech company tied every transformation job in dbt to business metrics like CAC and LTV, leading to consistent reporting across sales, product, and marketing for the first time.
"If data isn’t driving decisions, it’s just overhead."
- Business-first roadmapping: Data efforts are mapped to KPIs or OKRs, with clear outcome ownership.
- Cross-functional teams: Domains are composed of producers and consumers—aligned on shared definitions and SLAs.
- Governance within delivery: Quality, access, and lineage controls are automated within the engineering workflow—not added later.
This alignment shifts data from infrastructure to insight engine.
Choosing Your Strategic Approach
There’s no single blueprint for getting your data strategy right. Every organization brings its own mix of culture, maturity, and urgency—and your approach needs to account for that. Whether you’re centralizing governance, empowering teams to experiment, or balancing both, this section outlines the strategic models that drive sustainable transformation.
Use case: A European bank adopted a hybrid data strategy, setting a global vision centrally but empowering local branches to pilot their own domain-specific implementations—accelerating rollout while maintaining compliance.
"Strategy isn’t about control—it’s about clarity."
- Top-down: Executive leadership defines vision, mandates priorities, and drives alignment through governance. Effective in regulated industries or where data chaos is entrenched.
- Bottom-up: Teams pilot new processes/tools in pain areas, then scale best practices. Ideal for high-autonomy cultures.
- Hybrid: A strategic north star is defined centrally, while domains implement in ways that fit their context. This is the most sustainable model for growing orgs.
The goal isn’t uniformity—it’s coherence.
Embedding Governance by Design
For a deeper look at how AI can enhance foundational data processes like cleansing, check out our companion piece: AI in Data Cleansing.
Forrester research suggests that fewer than 10% of enterprises are advanced in their insights-driven capabilities, primarily due to inadequate data governance.
Data governance is often misunderstood as a blocker or a compliance checkbox. But in a modern data strategy, governance is infrastructure—it’s what allows teams to move fast without breaking trust. When embedded into workflows and enforced by automation, governance becomes a strategic enabler.
Use case: A healthcare technology firm embedded automated governance policies into their CI/CD workflows using dbt and Great Expectations—catching 92% of data issues before reaching analytics teams.
"If governance slows you down, you’ve designed it wrong."
- Declarative: Policies for schema, freshness, and access are encoded as code—not docs.
- Automated: Checks and tests run in CI/CD pipelines or at runtime—not in Excel sheets.
- Continuous: Alerts, observability, and anomaly detection make governance real-time and reactive.
When governance is treated like infrastructure, it scales with the org—not against it.
Platform Thinking: Build for Change, Not Just Scale
Throughput used to be the primary metric of data platforms—volume over velocity, centralization over adaptability. But modern organizations need systems that can shift direction quickly without losing structure. In a fast-changing landscape, the true test of a data platform isn’t how much it can handle, but how fast it can evolve.
Use case: A logistics platform rebuilt their data platform using modular services, reducing onboarding time for new datasets from 4 weeks to under 5 days, and enabling faster experimentation with ML models.
"Great platforms don’t centralize control—they decentralize confidence."
- Abstract complexity: Offer clean interfaces without hiding context.
- Enable trust: Deliver consistent SLAs across domains.
- Empower teams: Shift autonomy closer to the edge.

The Modern Data Stack, Rewired
In fast-scaling organizations, infrastructure decisions can either reinforce agility or quietly sabotage it. The modern data stack isn’t just a suite of tools—it’s the scaffolding that shapes how data flows, how fast teams ship, and how confidently leaders can act. When built with intention, it aligns with your operating model and unlocks velocity at scale.
Use case: A high-growth B2B SaaS company implemented an end-to-end modern data stack with OpenMetadata and Monte Carlo, reducing data downtime incidents by 80% and improving trust in product analytics across teams.
A composable, observable, and product-oriented data stack creates the foundation for trust, agility, and scale:
- Capture & Ingest: Kafka, Debezium, Fivetran, Airbyte
- Transform & Model: dbt, SQLMesh, Coalesce
- Orchestrate: Dagster, Prefect
- Metadata & Cataloging: DataHub, OpenMetadata
- Observability: Monte Carlo, Metaplane
- Quality & Contracts: Great Expectations, dbt tests, JSON Schema
- Access & Delivery: Looker, Hex, Hightouch
These aren’t just tools—they form the backbone of your data transformation journey.
Real-Time Is Now Table Stakes
Real-time data isn’t a bonus anymore—it’s the default expectation. In industries where customer experience, operations, and risk management are driven by up-to-the-second inputs, lagging pipelines aren’t just slow—they’re strategically dangerous.
Use case: A retail client moved from batch inventory updates to streaming Kafka pipelines, enabling real-time stock visibility across 2,000 stores and reducing stockouts by 15%.
"Real-time isn’t speed—it’s relevance. If data arrives late, decisions arrive broken."
- Stream what matters
- Use event-time and stateful joins
- Design for reprocessing and resilience
Modern use cases (fraud, personalization, operations) demand a system that reacts—not reports.
Data as a Product: From Theory to Operating Model
Treating data as a product isn’t just a shift in vocabulary—it’s a transformation in how teams operate, ship, and serve stakeholders. By thinking like product teams, data practitioners can build assets that are reliable, reusable, and measurable—creating value across the org instead of just delivering outputs.
Use case: A SaaS company productized its user engagement signals as a domain-owned, versioned data product. This fed marketing, customer success, and machine learning pipelines—driving personalized campaigns and 12% increase in retention.
“If your data doesn’t have an owner, a contract, and a feedback loop—it’s not a product. It’s technical debt.
And trust? That’s not a report. It’s a product you earn every day.”
- Ownership: Named product owners
- Contracts: SLAs, schemas, freshness expectations
- Interfaces: APIs, semantic layers, governed dashboards
- Observability: Feedback loops and usage insights
- Modularity: Composable, versioned logic
Where Are You on the Data Strategy Maturity Curve?
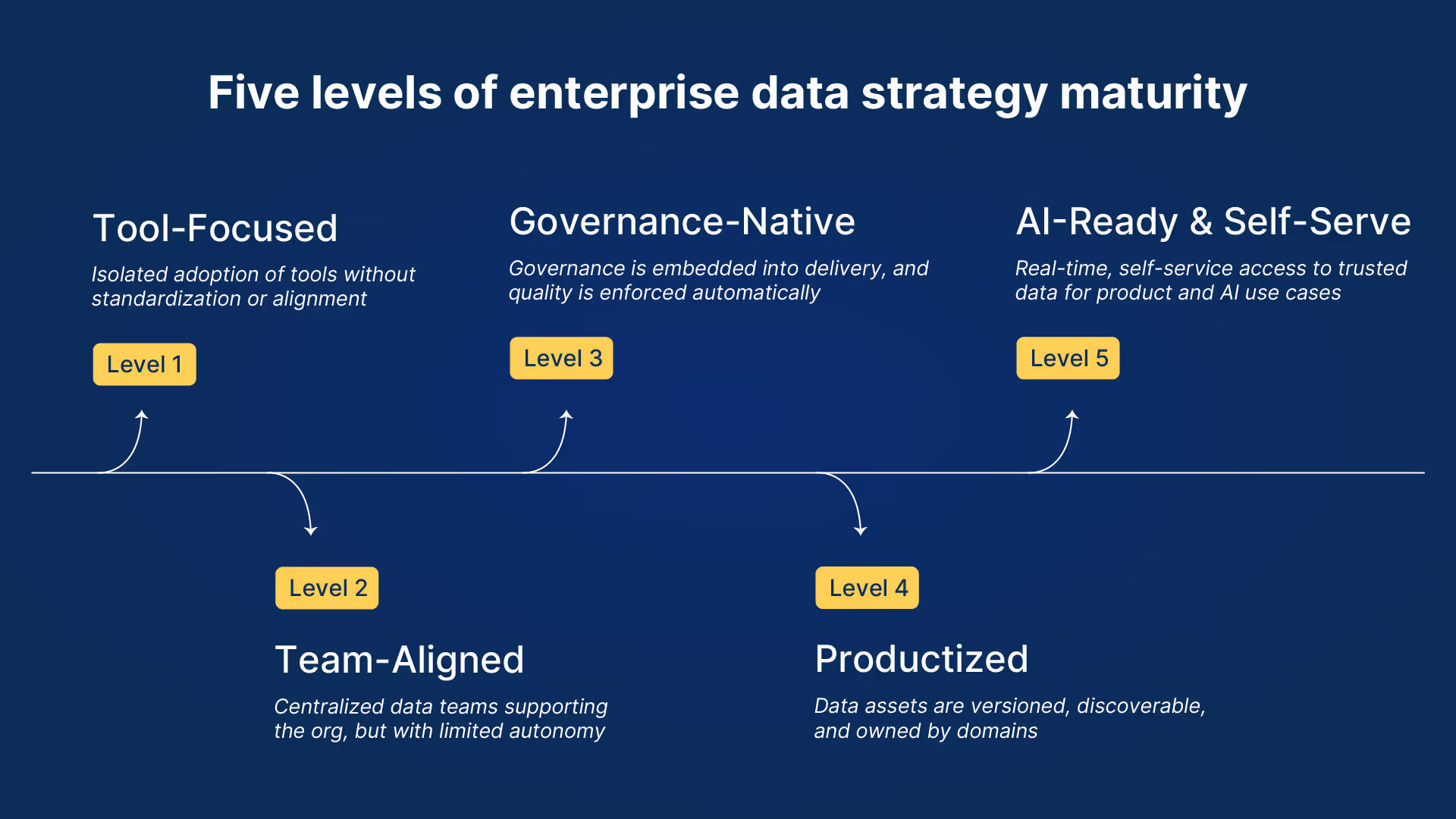
Not every organization is ready to leap to AI-driven platforms on day one—and that’s okay. What matters is knowing where you stand, and what to focus on next. Here’s a simple maturity model we use to help clients assess their current state and trajectory:
- Level 1: Tool-Focused
Isolated adoption of tools without standardization or alignment - Level 2: Team-Aligned
Centralized data teams supporting the org, but with limited autonomy - Level 3: Governance-Native
Governance is embedded into delivery, and quality is enforced automatically - Level 4: Productized
Data assets are versioned, discoverable, and owned by domains - Level 5: AI-Ready & Self-Serve
Real-time, self-service access to trusted data for product and AI use cases
Operational Principles for High-Impact Data Strategy
Effective data strategies aren’t just a checklist—they’re a working model for how teams ship faster, govern better, and scale smarter.
- Outcome-first: Every data initiative should ladder up to measurable business impact. Tie pipelines and models to revenue, retention, risk reduction, or efficiency—not vague goals.
- Governance-native: Embed quality, access control, and lineage into every step of your pipeline. If it’s not enforced in code, it’s not enforceable.
- Platform-powered: Build reusable infrastructure that abstracts complexity without limiting teams. Give every domain a launchpad, not a bottleneck.
- Product-driven: Treat data like software. Assign owners, publish SLAs, track adoption, and evolve based on feedback.
- Change-friendly: Build for versioning, rollback, and continuous improvement. Strategy should never be static—it should flex with the business.
Use case: A Fortune 500 manufacturer unified its enterprise data strategy around these principles, doubling the delivery speed of analytics use cases while improving compliance posture and cutting platform costs by 30%.
Above all, effective strategies evolve with the organization—and with the market.
The Ideas2IT Advantage in Data Services
At Ideas2IT, we don’t just implement data technology—we build strategic data capabilities that align with your business outcomes. Our data services practice is built around six key focus areas, each designed to help enterprises move from fragmented pipelines to intelligent data services and AI-ready, productized platforms:
- Data Strategy & Governance: Align data programs to business goals with clear ownership, policies, and operating models.
- Data Platform Modernization: Re-architect legacy infrastructure for scalability, interoperability, and cloud-native flexibility.
- Data as a Product: Define, build, and scale reusable, reliable, and discoverable data products with versioning, SLAs, and observability.
- Data Engineering: Build robust pipelines and workflows across batch and streaming, with modular design and governance baked in.
- Data Lakes and Warehouses: Design and optimize unified storage layers that power analytics, reporting, and ML training.
- ML Model Engineering: Accelerate experimentation and deployment with feature engineering, model pipelines, and performance monitoring.
We bring the strategy, architecture, and execution velocity needed to transform data into a business multiplier—at scale and at speed.
Conclusion: Data Strategy Isn’t Optional Anymore
In a world where data fuels everything from compliance to customer experience to AI, strategy is no longer a nice-to-have—it’s the backbone of sustainable growth. Without one, even best-in-class tools become siloed, underleveraged, or worse—sources of risk. With one, organizations unlock not just alignment, but acceleration.
Data used to be back office. Now it's the front office. Strategy is how you make the leap.
The next generation of enterprise leaders will treat data not as infrastructure—but as intellectual property.
If your data strategy isn't actively driving speed, trust, and scale—it's costing you more than you think. The difference between companies that innovate and those that fall behind will come down to how they treat their data.
Ready to rethink your data services foundation? Let’s build something scalable, governed, and outcome-aligned—together.