Credit: National Cancer Institute
According to the Congressional Budget Office, the pharmaceutical industry spent over $83 billion in 2019 on new drugs. With advances in machine learning in drug discovery, that’s only set to increase.
What does it really mean to explore the use of AI in drug discovery? Drug discovery, Machine learning, and large-scale applications for research and development are the keys to the next generation of healthcare. What does it really mean to explore the use of AI in drug discovery?
This guide will explore not only how AI is used in drug discovery but what’s on the horizon for this fascinating corner of development.
The Connection Between Deep Learning Models and Drug Research
Before we can look closer at applications of machine learning in drug discovery and development, we have to look at some fundamental concepts that set the stage for success.
A deep learning model refers to the heart of machine learning, which are neural networks. While neural networks have been around for a long time, computing solutions have caught up in a way where we can feed even more data into the network for processing.
The process of getting useful information out of data is at the heart of the mission for machine learning. Researchers must use the deep learning approach to unlock new insights.
Machine Learning Drug Discovery Insights
Application is at the heart of this aspect of technology; one of the most promising reports so far is that Seismic Therapeutic is using advanced machine learning drug discovery tactics to speed up its biologics pipeline.
Enhancing biological activity when each human body is so unique is the classic challenge of pharmaceutical research and development.
This element of machine learning can take on multiple forms, including the use of a support vector machine in order to speed up the classification of data. The faster that researchers can teach the computer to classify data, the faster the computer interface can return real information.
To build on this example, take a look at convolutional neural networks. This concept builds on support vector machines because it is also about classifying data.
The way it works is that the neural networks are broken up into various types, from recurrent neural nets all the way up to convolutional nets, which are computationally demanding and require a lot of power through GPUs to train the models properly.
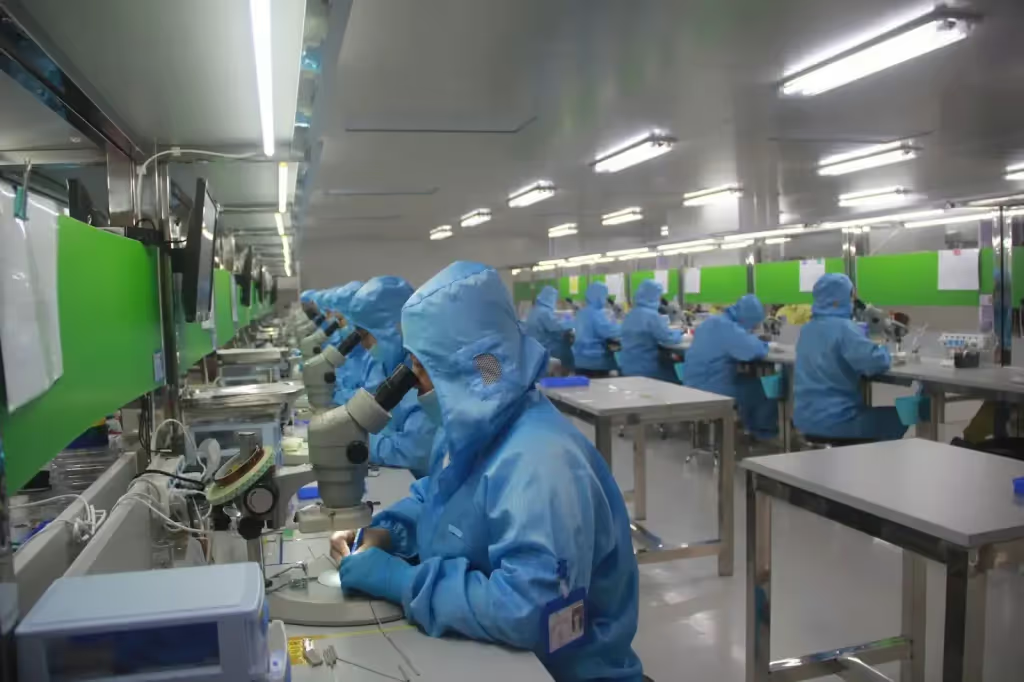
Credit: Glsun Mall
Technology Breaking Down Old Algorithms in Record Time
Here is a project that demonstrates exactly why machine learning drug discovery practices are so important. A team at the Massachusetts Institute of Technology discovered a new way to pair together chemistry and machine learning. The technique is called DeerBAR, where the BAR part stands for Bennett Acceptance Ratio. This ratio is well established as an algorithm to calculate binding free energy.
This algorithm is computationally expensive for several reasons: it requires multiple points of data between the drug molecule bound to a specific protein and one completely unconnected to a protein, as well as several states of time where the molecule and protein are “partially” bound to each other.
DeepBAR’s Impact on Drug Discovery Machine Learning
What makes DeepBAR transformative is that it not only speeds up the calculation speed, it paves a path for other applications in pharmaceutical research.
For example, processing the data quicker through artificial intelligence (AI) means these methods can transfer over to looking at chemical compounds for their drug candidate status or even drug repurposing.
The study is interesting enough that it warrants looking at it in full. The key takeaway is that DeepBAR measures binding affinity, an important metric in not only biological activity but the entire drug screening process.
If a molecule can’t bind to the protein, it isn’t a drug target viable for a clinical trial.
What Makes a Good Drug Candidate?
Speaking of drug candidates, it’s essential to also examine the primary metrics used to determine whether certain chemical compounds would qualify as drug candidates or not.
A paper published in the online edition of the BMC Neuroscience journal lays out the characteristics of what makes a lead chemical compound a good drug candidate: it must bind to the target, be bioavailable, and pass formal toxicity evaluation in animals.
The reality of research and development within the pharmaceutical sphere is that not every compound will lead to a true candidate for a viable drug. Without viability, a clinical trial cannot move forward.
It would not make any sense to pursue clinical trials of any kind without having the chemical compounds in place that truly address the intended mechanism within the body.
The Road Ahead for Machine Learning Techniques
Artificial intelligence modeling for drug discovery is an established practice that is only going to grow more important with time. Computing power isn’t unlimited, but the immense power at the fingertips of leading companies means that the public will see the benefits of this lifesaving research faster and faster over time.
There are plenty of studies to build interest in machine learning techniques, but working with a software development company could help you speed up the research curve for drug discovery. It is the next step to move from a conceptual overview to real-world execution.
The road to faster drug discovery is a matter of deep research, harnessing AI technology, and building software solutions that are usable for researchers, analysts, and other components within the research and development pipeline. Contact Ideas2IT to get started with Artificial intelligence and Machine learning for your drug discovery process.