With data management at the forefront of enterprise evolution, organizations are continually challenged to harness the power of their data efficiently.
Traditional database systems often struggle to meet the demands of today's data-driven enterprises, prompting the rise of innovative solutions like Snowflake Data Cloud.
Snowflake has revolutionized the concept of data warehousing by offering a scalable, cloud-based platform that seamlessly integrates data lakes and data warehouses into a single ecosystem.
As businesses increasingly rely on data to drive strategic decisions and gain competitive advantage, understanding what sets Snowflake apart from conventional DBMS becomes essential.
In this blog, we explore the distinctive features and capabilities of Snowflake Data Cloud that position it as a leader in modern data architecture.
But let’s start with a few basics.
What is a data warehouse?
A data warehouse is a centralized repository that stores integrated data from various sources within an organization.
Unlike operational databases that are optimized for transactional processing, data warehouses are designed for analytical purposes. They consolidate historical data and enable complex queries across large datasets, providing a structured framework for reporting and data analysis.
By organizing data into a consistent format, data warehouses facilitate business intelligence (BI) initiatives and support decision-making processes based on accurate, timely insights.
What is a data lake?
In contrast to a data warehouse, a data lake is a repository that stores raw, unstructured, and semi-structured data in its native format. It offers flexibility in storing vast amounts of data without the need for extensive preprocessing or modeling upfront.
Data lakes accommodate diverse data types and support scalable storage solutions, making them ideal for organizations seeking to capture and retain large volumes of data for future analysis.
Unlike traditional storage systems, data lakes prioritize accessibility and rapid ingestion of data, fostering innovation in data-driven applications and advanced analytics.
Difference between Data Lake and Data Warehouse
While both data lakes and data warehouses serve as essential components of modern data architecture, they differ significantly in structure, purpose, and usage:
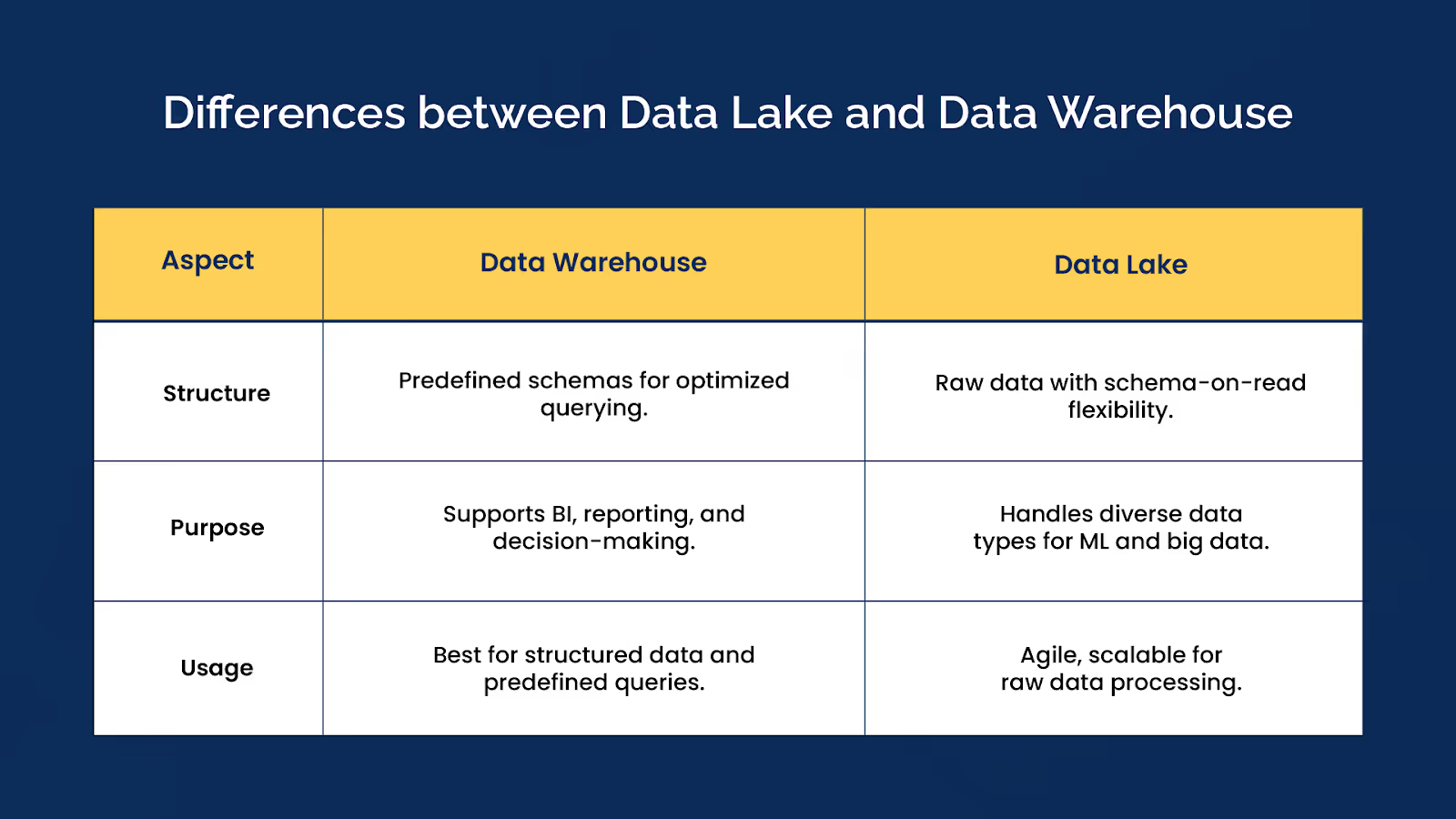
- Structure: Data warehouses structure data into predefined schemas for optimized querying and analysis. In contrast, data lakes store data in its raw form, allowing for schema-on-read flexibility.
- Purpose: Data warehouses are geared towards supporting business intelligence, reporting, and decision-making processes by providing curated, structured data. Data lakes are designed to handle diverse data types and support exploratory analysis, machine learning, and big data processing.
- Usage: Data warehouses are best suited for structured data and predefined queries, ensuring consistency and reliability in analytical outputs. Data lakes offer greater agility and scalability for storing and processing raw data, enabling organizations to adapt quickly to evolving data needs and emerging use cases.
Both data warehouses and data lakes play complementary roles in leveraging data assets effectively, with Snowflake data cloud bridging the gap between these two paradigms to deliver a unified data experience.
What is Snowflake Data Cloud?
Snowflake Data Cloud represents a paradigm shift in data management, offering a cloud-based platform that integrates data warehousing, data lakes, and big data processing into a single solution.
It is a cloud-hosted relational database designed specifically for building data warehouses. It operates on AWS, Azure, and Google Cloud platforms, combining the functionalities of traditional databases with innovative capabilities tailored to meet the evolving needs of businesses.
Built on its Multi-Cluster Shared Data Architecture, Snowflake provides a cohesive data experience, ensuring high performance and scalability. This architecture separates storage and compute, allowing organizations to independently scale resources based on workload demands, which optimizes both performance and cost efficiency.
Data loading into Snowflake is flexible, supporting both bulk and continuous loading processes, which is crucial for real-time analytics and data integration scenarios. Furthermore, Snowflake’s Time Travel and Fail-Safe features automatically handle data backup and recovery.
Organizations can define retention periods for database objects or select object types (Permanent, Transient, or Temporary) to manage storage costs effectively.
Snowflake’s cloud-based data warehousing capabilities extend beyond traditional systems by offering:
- Unified platform: Seamlessly integrates data storage, processing, and analytics, eliminating the need for separate systems for data warehousing and data lakes.
- Security: Ensures data protection with features like encryption, role-based access control (RBAC), and comprehensive auditing, ensuring compliance with industry regulations.
- Data sharing: Facilitates secure data sharing across organizations without data movement, enabling collaboration and monetization opportunities.
Snowflake Data Cloud empowers organizations to unify their data infrastructure, break down silos, and derive actionable insights more efficiently than ever before. It’s a combination of advanced architecture, security, scalability, and integration capabilities.
Snowflake Data Workloads
Snowflake Data Cloud supports diverse workloads tailored to specific data management and analytics needs:
- Data Warehousing: Snowflake provides a scalable, cloud-based platform for traditional data warehousing. It integrates with BI tools, supports complex SQL queries, and enables fast analytics and reporting.
- Data Lake: Snowflake acts as a unified solution for data lakes, accommodating semi-structured and unstructured data. It supports advanced analytics, machine learning, and exploratory data analysis without schema constraints.
- Data Engineering: It facilitates robust data engineering workflows with scalable compute resources. It supports ETL operations, data integration from various sources, and automates data pipelines for improved efficiency.
- AI and ML: It enhances AI and ML initiatives by providing a centralized platform for data storage and analytics. It integrates seamlessly with ML frameworks, supporting model training, testing, and deployment.
- Applications: Snowflake supports a variety of applications, from business analytics to real-time data processing. Its cloud-native architecture enables scalable application development and responsive user experiences.
- Cybersecurity: It prioritizes security with features like end-to-end encryption, fine-grained access controls, and comprehensive auditing. It ensures data governance and compliance, making it suitable for handling sensitive information securely.
Snowflake Data Cloud’s versatility across these workloads makes it a leading choice for organizations aiming to modernize their data infrastructure and leverage data-driven insights effectively.
What makes Snowflake Data Cloud stand out from other DBMS
The Snowflake cloud includes all the functionality of a data warehouse but with higher performance and better affordability through cloud computing. It is designed to manage every part of cloud-based data storage and analysis.

1. Automation & Scalability
Snowflake's cloud-native architecture offers extensive automation capabilities that enhance scalability, efficiency, and cost-effectiveness:
- Flexible Scaling: Users can independently scale compute and storage resources as needed, minimizing operational disruptions. Snowflake credits are billed with a minimum of 1-minute granularity, and storage costs are optimized through data compression.
- Auto-Scaling: Snowflake supports both vertical and horizontal scaling of computing resources. Auto-scaling automatically adjusts compute clusters based on workload fluctuations, ensuring optimal performance during peak periods without manual intervention.
- Auto-Suspend: This feature automatically pauses virtual warehouse clusters after a specified idle period, reducing costs by conserving compute resources. Active queries are completed before suspension to ensure seamless operations without impacting ongoing tasks.
By automating scaling and suspension, Snowflake minimizes the administrative burden associated with managing cloud infrastructure. Users can rapidly scale resources up or down, maintaining operational agility and cost-efficiency.
Snowflake's automation features empower organizations to dynamically adapt to changing data processing demands, ensuring efficient resource utilization and uninterrupted performance.
2. Support
Snowflake offers robust support for diverse data types and efficient data management capabilities:
- Comprehensive Data Types: Snowflake supports a wide range of SQL data types, including Numeric, String, Binary, Logical, Date and Time, Semi-structured, and Geospatial data. This versatility enables organizations to handle various data formats efficiently within a unified platform.
- Handling Semi-structured Data: It excels in managing semi-structured data, such as JSON, CSV, TSV, XML, and Parquet formats. These data types often require specialized pipelines for attribute extraction and structural alignment, crucial for modern data analytics and integration workflows.
- Data Sharing and Cloning: Snowflake facilitates secure data sharing among Snowflake users and non-Snowflake users. The Clone feature allows the creation of copies of data without additional storage costs, enhancing collaboration and data reuse.
- Performance Optimization: It incorporates features like result cache and data cache to accelerate data retrieval and reduce processing costs. These caching mechanisms improve query performance by storing frequently accessed data in memory, ensuring faster response times for analytical queries.
- Unified Data Handling: Snowflake's VARIANT data type enables seamless handling of both structured and semi-structured data within the same platform. This capability streamlines data integration and analysis processes, automatically parsing and storing data in a structured format for efficient attribute extraction.
Snowflake’s comprehensive support for diverse data types, efficient data-sharing capabilities, and performance optimization features make it a versatile and powerful choice for modern data management needs.
3. Compartmentalization and Concurrency
Snowflake DB leverages a multi-clustered shared data architecture that effectively separates its data storage and computation resources. This architectural approach enables Snowflake customers to benefit from faster response times and increased concurrency, allowing the system to handle multiple queries simultaneously with optimal efficiency.
Unlike traditional databases that often require separate instances to manage different workloads, Snowflake eliminates this need through its virtual Snowflake data warehouses. These virtual warehouses are isolated segments within the larger Snowflake cloud data platform, each functioning independently like a conventional data warehouse instance.
This setup not only enhances scalability but also simplifies administration by allowing organizations to manage diverse workloads without creating isolated silos.
Moreover, similar to traditional multiple data warehouse setups, Snowflake's virtual warehouses operate autonomously, ensuring that changes or issues in one do not affect others. This capability is particularly advantageous in dynamic environments where workload demands fluctuate.
4. Maintenance and Administration
At its core, Snowflake is offered as a data warehouse as a service (DWaaS) solution, a more specific solution apart from software as a service (SaaS) or platform as a service (PaaS).
This unique architecture allows organizations to deploy and manage data solutions without heavy reliance on database administrators or IT teams. There's no need for software installations or physical infrastructure setup, simplifying deployment and scalability.
Scaling projects, whether adjusting virtual warehouse sizes or cluster volumes, is streamlined in real time without manual intervention.
Snowflake further eliminates the need for manual server sizing, cluster management, and traditional database tuning tasks like indexing and calibration. Built-in resources handle these optimizations automatically, reducing administrative overhead and enhancing operational efficiency.
Routine maintenance, including server checks, upgrades, and software updates, is managed seamlessly by Snowflake’s dedicated team, ensuring system reliability and performance.
While Snowflake is not the only DWaaS supplier, its user interface rarely matches, especially with web browser support. For example, adding the SQL Server Management Studio allows you to access Microsoft Azure Synapse Analytics. It contains a suite of data integrations and Snowflake big data analytics capabilities that give you insights into data in your systems.
5. Security
Snowflake Cloud Data Warehouse prioritizes robust security measures to safeguard business information and ensure data integrity:
Snowflake employs industry-grade security across its infrastructure, including granular access controls and identity management using SCIM (System for Cross-domain Identity Management). Administrators can manage access through compartmentalized roles, automating user verification and enhancing security efficiency.
All Snowflake editions meet stringent standards for site and network access, ensuring secure communication via VPC/VNet. Security features like configurable session timeouts and key pair rotation bolster defenses against cyber threats, meeting diverse cybersecurity requirements.
Snowflake is compliant with global standards including Soc 1 Type II, Soc 2 Type II, HIPAA, PCI DSS, FedRAMP Moderate, and IRAP Protection, demonstrating adherence to rigorous security protocols and regulatory frameworks.
6. Shareability
Snowflake facilitates seamless data sharing across organizations, breaking down data silos and enhancing collaboration:
Snowflake enables real-time data sharing among multiple users, ensuring secure and efficient access to cloud data warehouses. With Snowflake's data-sharing feature, users can share tables, external tables, secure views, materialized views, and user-defined functions (UDFs) securely.
This capability eliminates traditional data silos, allowing diverse teams and departments to access and collaborate on shared data sets within Snowflake's secure environment. Businesses benefit from enhanced agility and collaboration in data-driven decision-making processes.
Key Takeaway
Snowflake Data Cloud is a versatile and powerful solution for modern data management. Its unique architecture supports seamless scaling, efficient data handling, and robust security measures.
For data scientists and engineers, Snowflake provides a flexible environment for advanced analytics and model building. Its scalable compute resources and support for complex workloads drive innovation and insights.
Seamless integration with various data tools streamlines the entire data science workflow, from preparation to deployment.
Incorporating Snowflake into your data strategy transforms your data warehousing, data lake, and data engineering efforts. Its powerful features and user-friendly design advance AI and ML applications, helping your business stay competitive in a data-driven world.
Ready to get started with Snowflake? Contact our professional team today to maximize your database adoption strategies and unlock the full potential of your data.